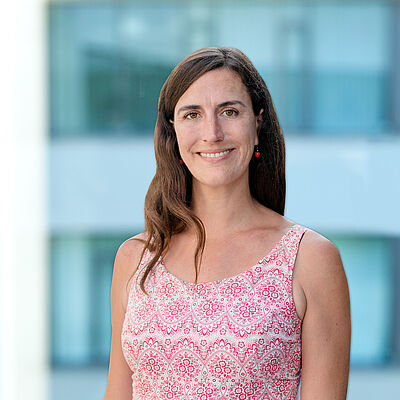
Asja Fischer
Institution: Ruhr-Universität Bochum / CASA
Research Hub(s):
Hub C: Sichere Systeme
E-Mail: Asja.Fischer@rub.de
Adresse: IB 3/153
Website: https://informatik.rub.de/ml/
Publikationen:
Characteristics of Monte Carlo Dropout in Wide Neural Networks Layer-wise linear mode connectivity Information Plane Analysis for Dropout Neural Networks Wasserstein dropout Marginal Tail-Adaptive Normalizing Flows Uncertainty-Based Detection of Adversarial Attacks in Semantic Segmentation DistriBlock: Identifying adversarial audio samples by leveraging characteristics of the output distribution Benchmarking the Fairness of Image Upsampling Methods Robustifying automatic speech recognition by extracting slowly varying features Copula-Based Normalizing Flows Thresholded Adaptive Validation: Tuning the Graphical Lasso for Graph Recovery Learning Sparse Codes with Entropy-Based ELBOs Uncertainty-Weighted Loss Functions for Improved Adversarial Attacks on Semantic Segmentation Efficient Calculation of Adversarial Examples for Bayesian Neural Networks Detecting Compositionally Out-of-Distribution Examples in Semantic Parsing Detecting Adversarial Examples for Speech Recognition via Uncertainty Quantification Leveraging Frequency Analysis for Deep Fake Image Recognition Uncertainty quantification with compound density network (Workshop-Beitrag) Infinite ensembles for uncertainty prediction (Workshop-Beitrag) Approaches to Uncertainty Quantification in Federated Deep Learning SmoothLRP: Smoothing LRP by Averaging over Stochastic Input Variations A Representative Study on Human Detection of Artificially Generated Media Across Countries AEROBLADE: Training-Free Detection of Latent Diffusion Images Using Autoencoder Reconstruction Error Towards the Detection of Diffusion Model Deepfakes How Sampling Impacts the Robustness of Stochastic Neural Networks